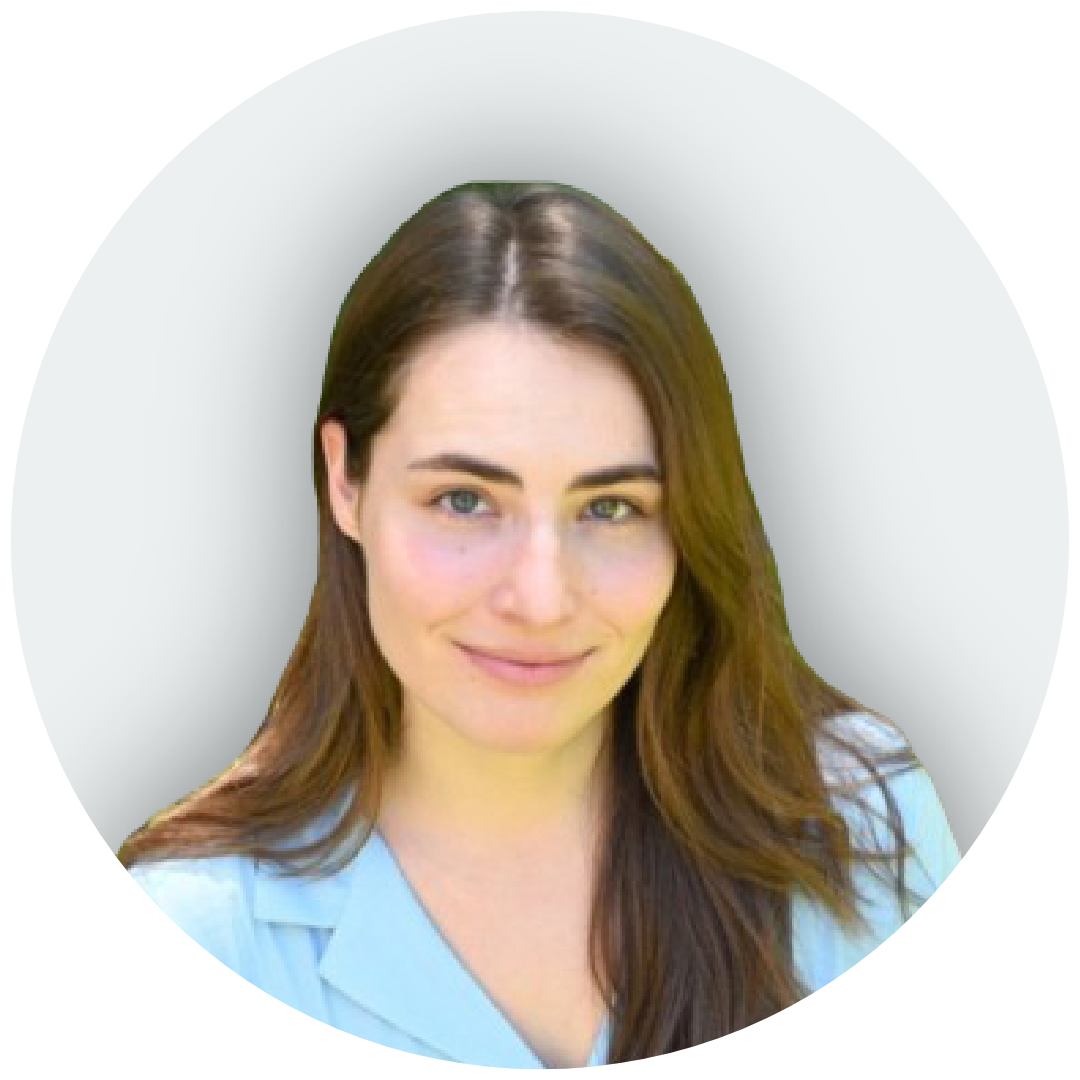
Hannah Lang
Team Lead, Digital Solutions
MineSense
Hannah is the Team Lead of Digital Solutions at MineSense Technologies and holds a Bachelor’s degree in geology from Cornell University and a Master of Science in economic geology from Queen’s University. Hannah draws on her geological background and knowledge of orebodies to support MineSense in unlocking the value of real-time sensor-based data analytics at the mine face to help mining operations increase their profitability and reduce their environmental footprint through mine-to-mill optimization.
At MineSense, Hannah has brought several projects to fruition including the development of machine learning algorithms to predict rock characteristics and the launch of MineSense’s client-facing data tracking and visualization tools. Her contributions culminated in the development of a portfolio of Digital Products, highlighting her creativity, innovation, and ability to collaborate across organizations.
Data Driven Mine-to-Mill Optimization
MineSense Technologies equips shovels and loaders with XRF sensors to provide real-time bucket measurements of material as it is being excavated. The primary purpose of the measurements is to predict the grade of material in each bucket. These grade predictions are integrated with the mine’s fleet management system to provide synchronous ore sorting to optimize recovery and reduce the quantity of waste processed by the mill — adding both economic and environmental value. Further investigation of these XRF spectra using key mine datasets and the application of techniques from machine learning has led to the discovery of relationships between extracted features of the spectra and other intrinsic rock properties. A noteworthy example is the classification of NAG and PAG waste, which can be used to sort trucks for optimal waste management with significant downstream environmental implications. Another is the classification of material by copper mineral speciation, which is consequential for mill processing performance. These associations are identified by engineering problem-specific feature sets, leveraging physical and geological knowledge as a starting basis to extract informative predictor variables from the XRF spectrum that are both directly and indirectly related to elemental composition. Care must be taken to account for confounding factors such as the spatiotemporal correlation of these properties, and these models can be validated by measuring the relationships between predictions and relevant mill processing KPI’s downstream. Here we discuss the methodology used in developing and validating a rock property prediction model with XRF data, as well as the broader implications for optimizing the entire mine-to-mill process.